Global Genes’ Inaugural Open Science Data Challenge expands known phenotype for Rare Neurodevelopmental Disorders
November 30, 2023
The Inaugural Xcelerate RARE Open Science Data Challenge ran from May 31 – August 31, 2023, with 132 researchers from across the globe participating. This challenge brought together clinical researchers, biostatisticians, and data scientists in a collaborative and competitive environment to make the best use of patient-provided data to address significant challenges to research and medical management for patients with rare neurologic diseases. Data from 27 different ultra-rare neurodevelopmental disease communities was contributed, including details on clinical and molecular diagnoses, symptom evolution and severity, and impact to quality of life.
One key task presented to challenge participants was to identify underrecognized symptoms or to expand the currently known phenotype of each of these rare neurologic diseases. Three winners were identified:
Best Approach (Combining RARE-X & External data): 3Billion Team (Won Chan Jeong, Bioinformatics Engineer; Kyoungyeul Lee, Chief Scientific Officer; Seoul, South Korea)
The 3Billion Team used a variety of methods, ranging from NLP to more traditional statistical approaches, and also employed multiple external datasets (Pubmed, OMIM, Orphanet, MGI) to find potentially unrecognized phenotypes. The team also suggested an approach to incorporate animal model data with human phenotype data
Best Open-Source Method to Benefit Rare Disease Research: Chong Lab Team (Jessica Chong, Ph.D., Assistant Professor in Pediatrics, University of Washington, Seattle)
The Chong team used a statistical approach called TF-IDF (Term Frequency-Inverse Document Frequency), a tool frequently used in search engine optimization. This framework was applied across the RARE-X and external datasets. The Chong Team’s approach provided a highly reproducible method of identifying phenotypes in rare disease across multiple data sources, and has already been contributed to an open source repository for other researchers to access and use
Most Innovative Approach to Analysis of Patient-Reported Data: Systems Biomedicine Team (Anaïs Baudot, Ph.D., Marseille Medical Genetics)
The Systems Biomedicine Team employed a statistical tool called “MultiXrank” which used an approach called “random walk with restart,” a classic statistical method often used to rank web pages and content. This method was used to compare the RARE-X data with Orphanet data, and then “walk” between the datasets. For diseases with Orphanet listings, this approach identified multiple likely novel symptoms for nearly every disorder included.
Another important challenge task centered around predicting a diagnosis based on symptoms, age of onset, disease severity, etc. Groups used machine learning algorithms to complete the challenge. In addition to the RARE-X data, this task included EHR data from 2 disease communities and external registry data from 2 disease communities. One winner was identified:
Best computational approach for predicting a diagnosis based on patient-reported data: Ambit Inc.’s Data and Analytics Team (Birnur Ozbas-Erdem, Ph.D., Vice President and Head of Analytics and Data Products, Ambit) This team’s rigorous approach significantly outperformed the base AI model, using neural networks and optimizing accuracy, precision, and recall.
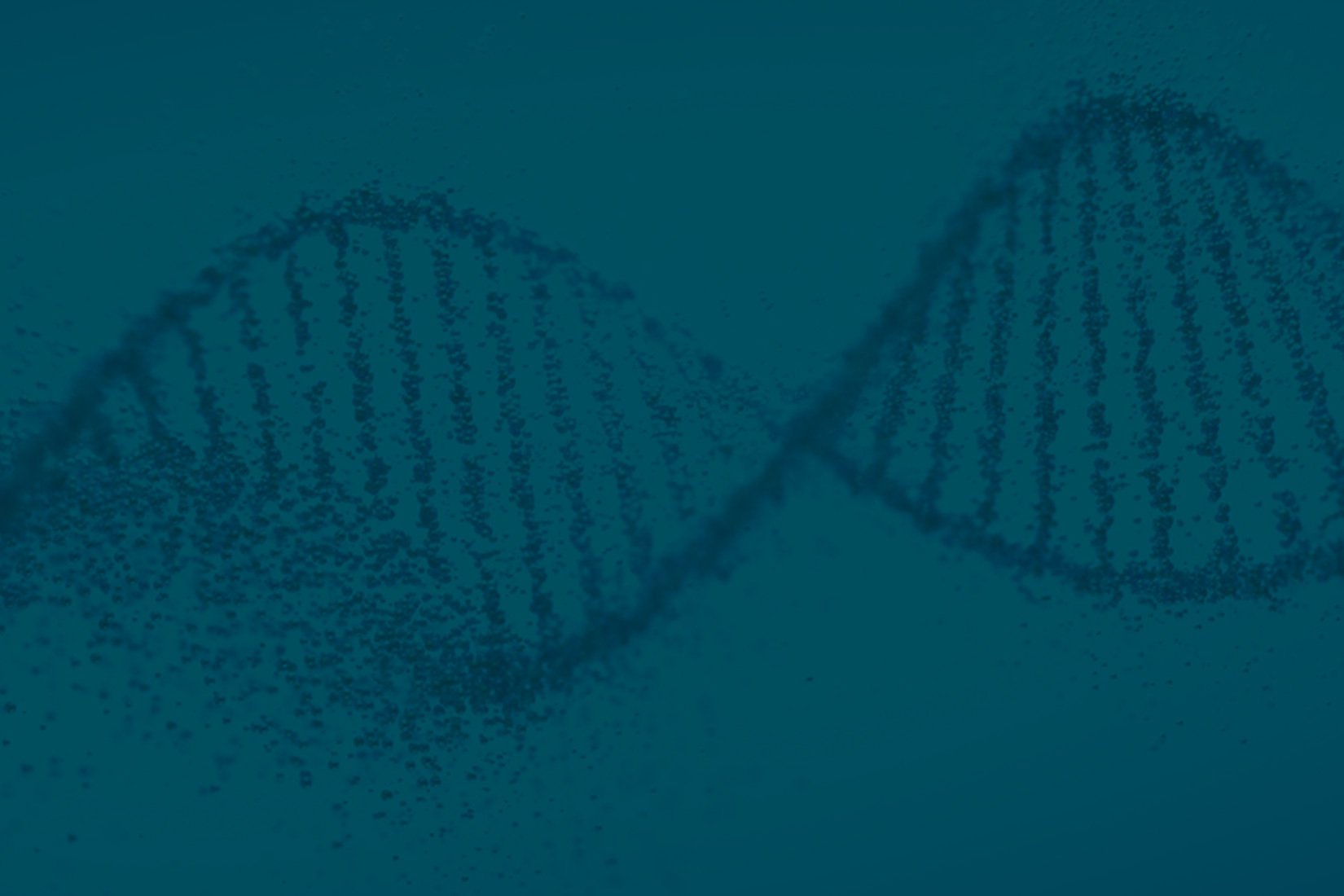
Stay Connected
Sign up for updates straight to your inbox.