Leveraging AI to Address the Neuropsychiatric Conditions Associated with a Rare Disease
November 30, 2023
Though many drug developers today are applying artificial intelligence and machine learning to accelerate and improve drug discovery, the results they produce, in part, may be limited to the quality of the data they are able to use. Nobias Therapeutics is not only using public and private data, but it boasts unique and proprietary access to one of the world’s largest and most diverse pediatric genomic data sets to drive insights into human biology. The company’s lead experimental therapy is a treatment for the rare condition 22q11.2 deletion syndrome, which is associated with a range of neuropsychiatric conditions. We spoke to Neil Inala, CEO of Nobias Therapeutics, about how AI is transforming drug discovery, the data Nobias is able to use to fuel its drug discovery, and the company’s experimental therapy for the neuropsychiatric conditions associated with 22q11.2 deletion syndrome.
Daniel Levine: Neil, thanks for joining us.
Neil Inala: Hi Danny. Thanks for having me.
Daniel Levine: We’re going to talk about AI, Nobias Therapeutics, and its efforts to develop a therapy to treat the neuropsychiatric symptoms associated with 22q11.2 deletion syndrome, a rare genetic disease that can include ADHD, autism, and schizophrenia as its symptoms. Before we talk about your experimental therapy, I wanted to begin with your use of AI and your sense of how this is reshaping the drug development process. AI seems to be becoming ubiquitous in drug discovery. Where do you think we are in its evolution?
Neil Inala: Yeah, thanks Danny. So, before I came to Nobias, I worked at Alphabet for 10 years in a bunch of areas where AI is critical to the future of the company, and this includes Google Ads and Cloud, but also the healthcare group Verily, and Waymo, which we all know wouldn’t exist without the use of advanced modern AI methods. I saw AI techniques integrated and embedded into all kinds of things you can’t even imagine without deep immersion in a specific problem space. If we consider the drug development problem space, I think we’re still in the very earliest days of the impact of modern AI methods on drug discovery. To get a sense of scale, you can compare the adoption curve of AI to that of networking and the internet. Alohanet was created in 1971 to connect the University of Hawaii computing facilities, and now 50 years later, we’re still exploring the impact of Ethernet TCP/IP and the internet on our society. I think AI will reshape every part of drug development and right now there is a lot of focus on the discovery phase, but it’s going to get integrated into medicinal chemistry, trial design, even things like patient monitoring and assessment. Nobias Therapeutics is specifically working on accelerating that adoption curve, in particular using modern machine learning and AI techniques to number 1, rapidly get to a better understanding of the most effective biological targets, and number 2, to get a better handle on the likely efficacy that drugs will have at the end of that development pipeline.
Daniel Levine: When you think about the impacts of AI on drug discovery and drug development, do you see this as just another tool to make things better, faster, cheaper, or is there something transformational about AI? Can it enable the discovery of drugs that would not otherwise be possible?
Neil Inala: Yeah, of course, better, faster, cheaper will always be a goal, but the real promise of AI is what it can do for us that will completely change how we think of what’s possible. I like to think of it almost like Maxwell’s Demon, which is a concept from physics. Imagine your smartest friend shrunk down and injected into the deepest crevices of every question you want to answer. I mean, even better, imagine a thousand or a million of them and they don’t get tired and they work 24/7. Okay, you thought modulating protein target X will help with this disease, but did you consider what happens with vesicular trafficking or with post-translational protein modifications? Can we dive more deeply into transcription enhancers or what happens with tissue specific expression? And all that’s just on the target side. Those are the kinds of questions we would expect an AI to really start to help with and transform how we answer these questions. Another huge advantage is that AI lets us ask these questions where we don’t really know the answer right now and we don’t know necessarily how to get to it. If you have enough data, it can infer things that you would only get if you sat in a basement bleary-eyed, staring at data for a hundred years, for example, building up hypotheses about protein structure based on looking at thousands of example structures as it was done with AlphaFold.
Daniel Levine: Nobias has built a suite of AI tools. What’s the range of things you’re doing with it and how are you using the technology?
Neil Inala: Well, that’s a very interesting question. From the beginning, we didn’t really set out to take just a single technique and build a company around it. Instead, we wanted to take a step back and broadly ask that question of where is AI going and how can we use it to make drug discovery more efficient? And the answer we came up with is let’s build a suite of AI tools and a way to put them together and take them apart in a flexible way to ask biological questions and generate biological insights. In fact, let’s atomize those tools to a very granular level and develop a facility that lets us create tools out of sub tools and recombine them in sophisticated ways. Some of your listeners may be familiar with 3-D modeling tools or non-linear video editors that are node-based. These let you take small bits of functionality and recombine them in different ways. We’ve basically done the same thing, but with AI tools that ask and answer different questions. For example, we built a tool, we call it the Symptom Expander, that looks at a host of related symptoms and develops hypotheses about what genes may connect them all together. Another example is a tool that given a specific known disease extrapolates from known drug treatments, key proteins that are involved in that disease. And we have dozens of other tools like this that we’ve developed. We’ve even built molecular modeling tools that use sophisticated mathematical methods together with neural networks to rapidly tell us binding affinities between proteins and small molecules. So, we’ve really tried to look at the end-to-end for the discovery process and figure out where can we inject AI and ML to make that process better and help us answer difficult and more complex questions.
Daniel Levine: My sense is that how good an answer you get from all of this is somewhat dependent on the data that’s being used. What’s the data sources you’re using to drive drug discovery and what’s the range of inputs and what’s the output?
Neil Inala: Yeah, that’s a really great question. Data is of course incredibly important for anything you want to do in the AI space. It really determines how good your tools are, and as we know, most of the modern advances in large language models, ChatGPT and so on, really come from answering question, basically providing huge amounts of data. In our case, we have unique access to a dataset that was assembled by a large hospital partner of ours in Philadelphia. It’s the largest pediatric genomic database in the world with genomic data from more than 130,000 patients, including electronic health records, lab data, imaging data, and pathology data, and 50,000 genomes from parents and siblings. Our platform has also ingested nearly 30 other curated private and public data sets, and these form a complex and dense interconnected web of information. On top of that collection of databases, we’ve built what’s called a graph convolutional network, which can find connections you might not otherwise expect based on closeness in a type of conceptual space called a latent space. We also have an ability to ring-fence data so that we can incorporate partner data for work with that partner only. But in terms of outputs, we actually don’t see our AI tools as spitting out an answer so to speak. They actually enable a rapid iterative hypothesis generation approach that we feel is best done with human experts in the mix with the AI system.
Daniel Levine: So, it’s kind of a design, test, build cycle.
Neil Inala: In software development, people may have heard of what’s called a ‘REPAL’ that stands for ‘Read Eval Print Loop.’ For us, it enables that kind of question. A lot of us come from the computer science area, and so being able to rapidly iterate like that really makes the difference between being productive and being very slow. Sometimes in a software development lifecycle, you can do that iterative process multiple times in a minute, and the difference between doing that and having a very complex process where you got a kind of ‘think about it’ question and then launch an experiment and eventually get an answer, and maybe pipeline all those in parallel, it’s just very complicated and very different from the approach we’re trying to enable for the drug development process.
Daniel Levine: And is the AI system able to learn from each of those iterations?
Neil Inala: Yeah, that is a really interesting question. So not to get into too much data from my past history, but Google gets a lot of information when you type things into a search box and if you type something, you look something up, you click on something else and then you type in something more that is a sort of a back and forth with the system and you can learn a lot from that process whether somebody thought you had good results, bad results, whether you are honing in on a particular topic or you’re expanding what you’re trying to do. And for us, when that’s one of the things that we’re building, we haven’t quite built that part yet, but basically we can watch what happens as we interact with that system and say, is there a part where we need to build additional tooling, where we need to be able to dive more deeply and answer these questions in different ways? So we think that’s going to expand and be more and more exciting over time.
Daniel Levine: So, this may seem like a strange question, but if it’s an iterative process, how do you know when to stop iterating?
Neil Inala: Well, I mean I could make up something about you look for an algorithmic approach where you reduce distance to a particular target and you measure how rapidly you’re reducing that distance, but the reality is it’s a little bit of a gut feel right now. There are some things I don’t want to quite get into in a public forum, but we do have some techniques that are based on theory improving that do let us answer ‘are we close to knowing a lot of the stuff we need to know’ and we try to deploy those as well.
Daniel Levine: As I mentioned earlier, your lead experimental therapy is a treatment for the neuropsychiatric conditions associated with 22q11.2 deletion syndrome me. Can you explain what that syndrome is?
Neil Inala: Yeah, I’ll do my best. So, 22q11.2 deletion syndrome. It goes by many names including DiGeorge syndrome, velocardiofacial syndrome, and others. And it’s a deletion on chromosome 22 of 55 or so genes. And as you know, we have a bunch of chromosomes in the human body. Some of them are more—I guess they’re all critical—but some can have mutations and modifications that still allow children [and] adults to develop. In the case of 22q11.2 deletion syndrome where they’re missing these 55 genes, the deletion alters the development of the third and fourth pharyngeal pouch during embryonic development, among many other things. This leads to heart defects, poor immune system function, a cleft palate, and related complications like low levels of calcium in the blood and many other issues. Many of these can be corrected surgically at birth or a bit later, but around the time they reach school age, they start to experience other stresses and in many cases they start to manifest certain neuropsychiatric symptoms like ADHD, anxiety, autism type behaviors, and so on. And so even though the physical items I mentioned were corrected surgically, these neuropsychiatric symptoms require other approaches. Often what’s used are conventional stimulants and things that are used in people who have only ADHD or only autism and so on, but they may not work as effectively in these children who have this complex presentation. So that’s one of the reasons we developed this drug and in fact, that’s what we’ve been testing in our recent clinical trials.
Daniel Levine: Yeah, it’s interesting to see this because unlike areas such as cancer where we’ve grown very accustomed to precision medicines, psychiatry has been an area that we’re only now seeing the development of precision therapies. This is a genetic condition. Do you know if there’s anything particularly unique about ADHD, schizophrenia, or autism when it’s caused by 22q11.2 deletion syndrome relative to other forms of these conditions?
Neil Inala: Yeah, that’s a very interesting question. At Nobias we like to think that rare diseases can provide insight into more common diseases, and those common diseases may not be a singular disease, but could be a set of more rare conditions that all manifest in a similar phenotype. So 22q11.2 is an example of that. We know that the neuropsychiatric symptomatology of these patients is one of what’s called syndromic ADHD, or ADHD as part of a syndrome, in this case with comorbid autism and/or anxiety. And we think that this is caused by a chromosomal deletion and, in particular, variants in the what’s called MGluR or metabotropic glutamate receptor gene family network. This signaling network plays a role in several neuropsychiatric conditions, and we think that rescuing MGluR signaling with an MGluR activator like NB-0011 will not only help 22q11.2 patients, but may be used in other indications with variants in that gene family network.
Daniel Levine: Your experimental therapy is NB-001. How was this discovered?
Neil Inala: There is definitely some history to the study. NB-001 is actually a drug that was originally developed by Nippon Shinyaku, a Japanese company, for vascular dementia and was brought through phase 3 trials by them, but shelved for lack of efficacy. Our chief scientific officer, who is a guy named Dr. Hakon Hakonarson, who has got a fascinating history, I’d love to go into that at some point, but he was looking for a drug that was known to be sort of a modulator of these metabotropic glutamate receptor networks and generally safe and usable for certain neuropsychiatric purposes. And so he found this drug and contacted the company, licensed it from them, and we decided to take that forward and repurpose it for this trial and this purpose.
Daniel Levine: How well understood is the mechanism of action of NB-001?
Neil Inala: Yeah, there’s a lot of consternation that has gone into figuring that out. Of course, Nippon Shinyaku did a lot of trials in animals and cell lines and other things to figure out what’s going on. The truth is that the exact mechanism of action is something that we have our engineering team working on and they’re developing some pretty interesting insights. And I think that some of these things are only discoverable with the use of tools like we’ve built. It’s pretty amazing that it’s more than just an MGluR activator, but I won’t go into much more than that. But what we can say is that NB-001 is believed to be an activator of MGluR or metabotropic glutamate receptors, which are a family of what are called GPCRs, or G-protein coupled receptors, that kind of are involved in the modulation of synaptic transmission and neuronal excitability throughout the CNS, or central nervous system.
Daniel Levine: What’s known about the safety and efficacy of the candidate from studies you’ve done today?
Neil Inala: So, we do know that the drug at this point is very safe and safety was the primary outcome we were measuring in our recent phase 2 trial. We had no grade 3 or above adverse effects in our phase 2 study, and this mirrors prior clinical experience with the drug by Nippon Shinyaku and others in terms of efficacy. We recently released our public top-line data on that from our phase 2 trial. We used an endpoint called CGII, which is Clinical Global Impression of Improvement, and in our case, it was a scale of 1 to 7 where 4 is a baseline, and really you’re looking for improvements, like a 3, 2, or 1, which indicate getting better and better on the scale. And in general, we saw improvements of between a third to a half of a point, which is what we’ve seen as clinically significant in other similar kinds of trials. Specifically, we saw these outcomes in our full analysis set, which is the most conservative set. That’s all comers who came into the trial. We saw an improvement of 0.36 compared to placebo. And then in subgroups, our per protocol set as well as in groups defined by certain impairment on some of these scales, ADHD, autism, and anxiety, we also saw really interesting improvements comparable to those in the full analysis set.
Daniel Levine: You’re also developing a second experimental therapy. This is NB-002 for vascular anomalies. This is in preclinical development. Are these genetic in nature and how serious a health threat are they for people with a condition?
Neil Inala: Yeah, they’re definitely genetic and the result of a variety mutations, but they generally fall into two key pathways. I mean, all of these are basically, you could think of them as cellular stress and response pathways, cellular growth and so on—a lot of connections to how cells respond to insults and growth signals and other things that happen in the body. And there are often a lot of connections back and forth to cancer signals. But I mentioned these two key pathways. One is called PIK3CA, and the other is called the Ras-Raf MEK kinase pathway. And these are also called rasopathies. So there are several types of vascular anomalies. However, we’re focused on a set that are called—you could call them complex lymphatic anomalies, and they’re considered rare, in some cases congenital, in other cases somatic, which means that they arose later from a random mutation in some set of cells. And children who have these complex lymphatic anomalies, they develop benign cysts, benign in the sense that they’re not malignant like in cancer, but these are called lymphatic malformations and often in their lymphatic system, although they can occur also in the arterial-venous system. These cysts can grow into the child’s bones, their connective tissue, and organs, damaging them and severely disabling the child. They can also cause leakage of lymphatic fluid into certain body compartments, including around the heart and lungs make it hard for you to breathe or hard for your heart to beat. And unfortunately, mortality rate for some of these can be as high as 40 percent. So it’s quite serious. And there are no FDA approved drugs for treating these.
Daniel Levine: How did Nobias come to develop NB-002?
Neil Inala: Well, that’s an interesting story with an interesting background. So our hospital partner is a center of excellence for treating children the world over. And so definitely there are children who come in who have some of these anomalies. There was an example of a kid who came into the hospital who they gave him the name Daniel in some of the public stories about him. I don’t think that’s his real name, but he was an athletic kid, a soccer player, and a runner, and he grew up being fine, but then at some point he developed what we call the somatic mutation. So things just started growing and overgrowing around his heart and lungs and other areas. And when he came into the hospital, he was basically in a wheelchair and not doing very well, and they had already tried one drug that is sometimes used in these things, but that drug only works in 50 percent of the cases and it didn’t work on him. So, our chief scientific officer, Dr. Hakon Hakonarson, developed a cell-free DNA sequencing technique where he was able to extract the lymph fluid from the lungs of this patient. And also, there was another patient who was an adult who came and had similar issues. And from them [he] discovered through whole exome sequencing that there was a gene that was stuck on. It was constantly signaling to these cells to grow. And when you’re telling lymphatic cells to grow, they can kind of make that lymphatic network a little bit looser so that fluids start to leak out. This gene that was stuck on was upstream of a gene called MEK. And so they decided to use a known class of drugs called MEK inhibitors, and MEK inhibitors are mostly oncology drugs. I’ll get into that in a second. But he basically decided to try this out on zebrafish and cell lines that modeled in some ways the symptoms that they were seeing in these patients. And [he] then discovered that basically it was doing an amazing job of fixing those problems. And in particular, it remodeled the lymphatic system in these zebrafish, which is something that had not been seen before. So after applying for a compassionate use exemption with the FDA, they gave this child the drug, and he pretty immediately started to get better and at some point was able to get out of the wheelchair, and now it’s like five years later and he’s doing fine. And they’ve also been able to treat a few other children with similar diseases who are affected in the same way. And they’re all showing, or not all, but a good portion of them are showing really amazing improvements. So that’s where we decided to take this same class of drugs and develop a particular molecule because the drug that they were using is considered an oncology drug. And in oncology you have some pretty severe side effects that you wouldn’t necessarily want to accept for a child who’s going to be on this drug chronically for most of their lives. So, we looked at developing something that would have the same efficacy as what they were using, but that would have a better safety profile and also a better business profile from IP point of view and so on. We now have that drug in hand and we’re readying our next regulatory steps to take this through a phase 2 trial.
Daniel Levine: There’s not a clear connection between vascular anomalies and 22q11.2 deletion syndrome. How does Nobias think about building a pipeline and what constitutes a condition you’d pursue?
Neil Inala: We ground a lot of the work we do in what data we have access to and what we can generate from our tools. So I’d mentioned before this database we have, which is an amazing, incredibly rich resource that guides a lot of what we can do. And if we can’t pull something from that, we look at what are some of the other 30 or so databases we’re connected to and what insights can we gather based on that. In a sense, I’d mentioned before that we’re focused on rare diseases and how these rare diseases can act as lenses to give us insight into more broad diseases, more commonplace diseases. Well, that’s sort of the same approach we take with querying the database or multiple databases. We look at ‘is there a direction we can go where it opens up series of biological insights about something that we can turn into related families of drugs?’ In the case of the 22q11.2 deletion syndrome and vascular anomalies that we have initially developed drugs for, those come from querying that database using a variety of methods and in some cases, prior to when we developed our AI tool suite, but they still came from using algorithmic methods, biostatistics, biostatistical methods, and other mechanisms to really look for unusual connections that lead to these biological insights. So we are guided by that information that’s in the database, how we can construct a series of biological insights that tell a story about what’s happening biologically and how we can turn those into something that’s a drug. And finally by what our partners suggest because we have a lot of capabilities that we’re discussing with potential pharma partners and they have their own interests guided by all kinds of considerations. They may be into fibrosis or they may be looking specifically at neurological disease and so on. And those are the kinds of things that we use to guide the development of our pipeline.
Daniel Levine: You mentioned partnering. What’s the company’s partnering strategy? What would you seek in a partnering opportunity?
Neil Inala: So, we have, in the collection of our platform and datasets together, an incredibly strong capability to generate a volume of biological insights very rapidly. And what we’re focused on is turning those into a series of products. In most cases, those products will ideally be drug programs, and in some cases they may be just IP. In other cases, they may stop at phase 2 and so on. But we are really looking at a lot more opportunity than we can or really should advance just on our own. So we see that as a partnering strategy as encompassing three key phases. This includes target discovery, clinically validated programs, and new indications or repurposing. From a target discovery point of view, we’re looking at partnering with larger companies that are interested in novel target identification, and we have access to genetic data from nearly 14,000 diseases. So, the playing field here is quite large and exciting. We’re also looking for under-clinically validated programs. We anticipate advancing certain programs through a clinical proof of concept and then partnering those with larger companies with late stage and commercial expertise in that particular disease area. And in terms of new indications and repurposing, one of the advantages is that we have also built, in addition to these target discovery tools, we built a lot of chem informatics tools from scratch that use moderate ML methods and really unusual sophisticated mathematical techniques to answer really deep and detailed questions about efficacy and potential reuse. So we can quickly identify possible additional indications and or repurposing opportunities for shelved drugs. And that would be the third leg of our current partnering strategy.
Daniel Levine: And how is Nobias financed to date and how far will existing funding take you?
Neil Inala: So, there’s a little bit of history there. We were funded by Domain Associates originally and Medical Excellence Capital. Domain is known for doing company formation. And our chairman of the board, Brian Halak, was VC at Domain Associates. So he was looking around at ways to take modern genetic databases, particularly some of the really large ones that are at a variety of institutions and coupling those together with advanced analytical tools, particularly including AI and ML techniques. So, he contacted our co-founder, Dr. Hakon Hakonarson, and they decided to get together and take a couple of these programs that we have now, lymphatic anomalies program and the 22q deletion program and start with those as clinical trials and developing those molecules, and then bring on people as well who had expertise in AI and really development of AI-based systems. And of course, that’s where I come in and we spent the last couple of years building that stuff and we were financed by this combination of Domain Associates, which put in the initial capital and then subsequent additional capital infusion by Medical Excellence Capital. And then we’re now post phase 2 trial. We’ve just received a small bridge from our current investors that will sustain us for a little while until we are able to bring in our next big round of financing. And we’re working on that fundraising right now.
Daniel Levine: And it’s been a tough environment for biotechs to raise money. There’s been a bit more investor interest in companies with AI platforms, but what’s the discussion with investors been like for you?
Neil Inala: Well, as you know, it’s a very tough time. Many biotech companies were being held for something like a 70 percent discount on their actual cash assets. So the market is thinking, well, I guess these companies are going to destroy value. And that of course cascades out to the market beyond the public companies and into the VC space and so on. So we of course think we’re going to add accretive value and very rapidly get to a point where we have an amazing set of assets, but it’s an uphill battle for all companies that are in this space trying to raise capital right now. We do have a very supportive board, and as I mentioned, they’ve put additional money into the company and they’re excited about what they’re seeing come out of our platform. The data for our 22q program and our multiple other potential drugs that are in our pipeline, that money kicked off an effort to bring in our first large institutional round. And we’ve spoken now to a lot of companies. I think no one really believes it’s going to be an easy task right now. You are going to have to speak to probably dozens, maybe even a hundred or more VCs just to get that next term sheet. But our board has been phenomenal in helping us with that. They’ve amplified our investor outreach through their connections and really helped us with a lot of introductions and ideas for how to develop what we’re trying to do. We know these VCs have got their hands full, keeping their current portfolios afloat, really looking at what’s happening in the markets, forecasting where things are going to go, particularly with legislative acts, and a huge amount of private companies coming to them asking for fresh capital. So, we know all that. We are having good conversations. It seems like a lot of investors are focused on value creation and are really probing deeply to understand what our platform can deliver and differentiation in our clinical and preclinical programs. We’re in early days still, but we’re confident in the progress we’ve made and the way that we’ve de-risked our portfolio and our platform, and the opportunities ahead that will enable us to get this raise done.
Daniel Levine: Neil Inala, CEO of Nobias Therapeutics. Neil, thanks so much for your time today.
Neil Inala: Thanks very much, Danny. I really appreciate it.
This transcript has been edited for clarity and readability.
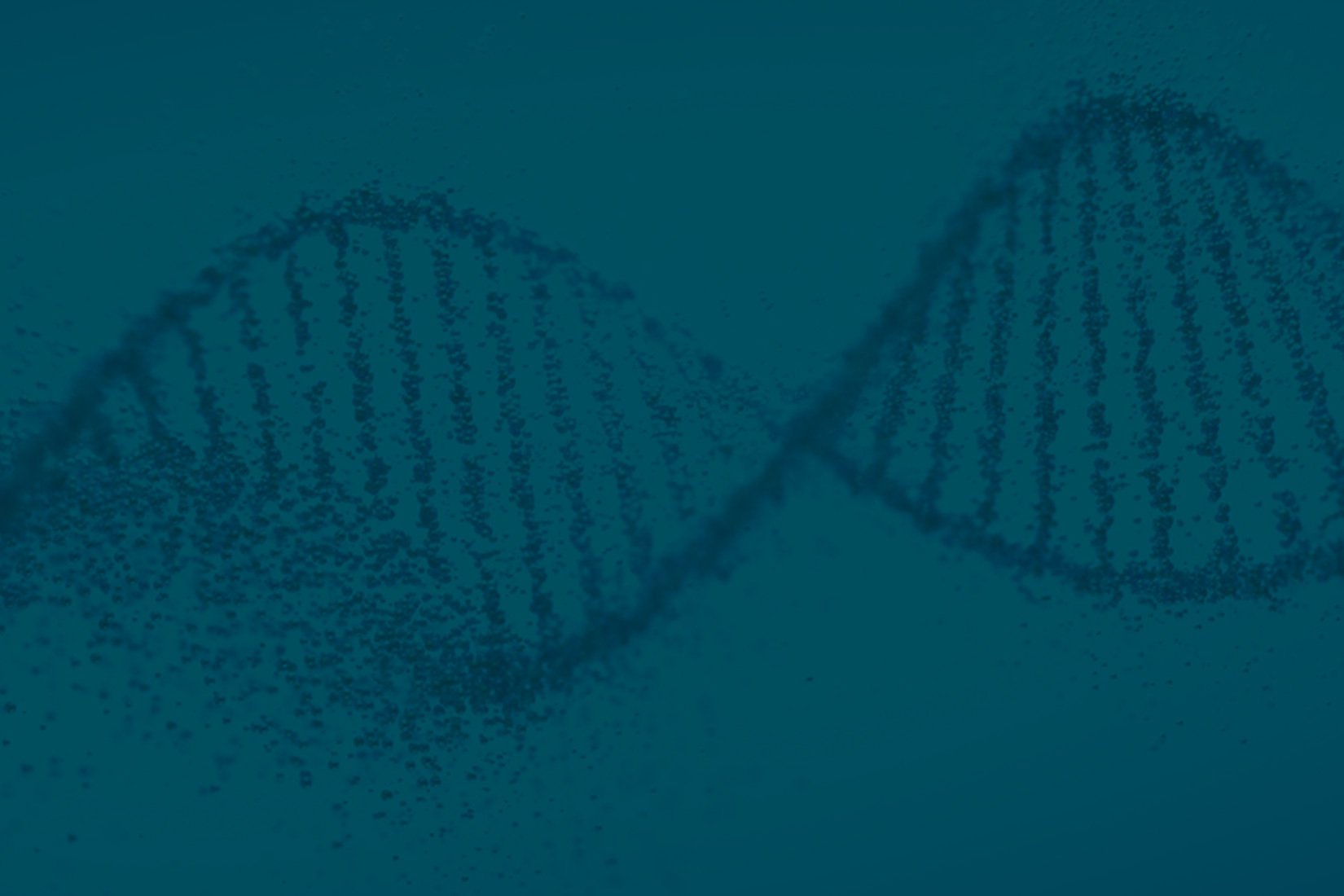
Stay Connected
Sign up for updates straight to your inbox.